1. Pavement Image Data Set for Deep Learning: A Synthetic Approach
This research aims to explore the viability of using synthetic pavement image data to train convolutional neural networks (CNNs) for automated pavement crack detection. A procedural approach of generating synthetic pavement crack image data is proposed. The results indicate that training a crack detection model using only synthetic data can reach almost the same level of accuracy as using the real data.
1) Real 3D image 2) Synthetic 3D image
Fig 1. 3D image examples
Research paper link: https://ascelibrary.org/doi/abs/10.1061/9780784483503.025
2. Automated Pavement Crack Detection with Deep Learning Methods: What Are Main Factors and How to Improve the Performance?
This research discusses the main influencing factors that affect the performance of DL models in pavement distress detection and aims to explore possible ways to improve the model performance. An open-source pavement image dataset and three promising object detection methods were selected for model development and evaluation. A series of experiments were conducted with different settings of model structures and data manipulation. According to the experiment results, selection of detection methods, data size, and annotation consistency have significant impact on detection performance.
3. Improved One-stage Object Detection Model for Pavement Distress Detection Using 3D Pavement Surface Images
An improved one-stage detection model is proposed by introducing GhostNet into the YOLO architecture. A series of experiments are conducted using the developed dataset. According to the results, the proposed model is able to achieve an overall F1 score of 0.81, which demonstrates a great potential for distress classification and quantification with high-resolution 3D images.
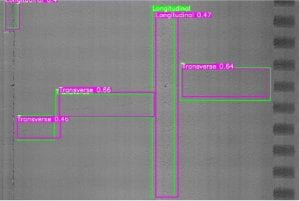
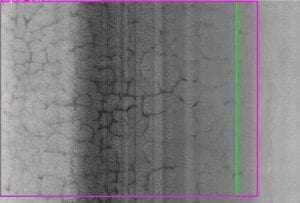